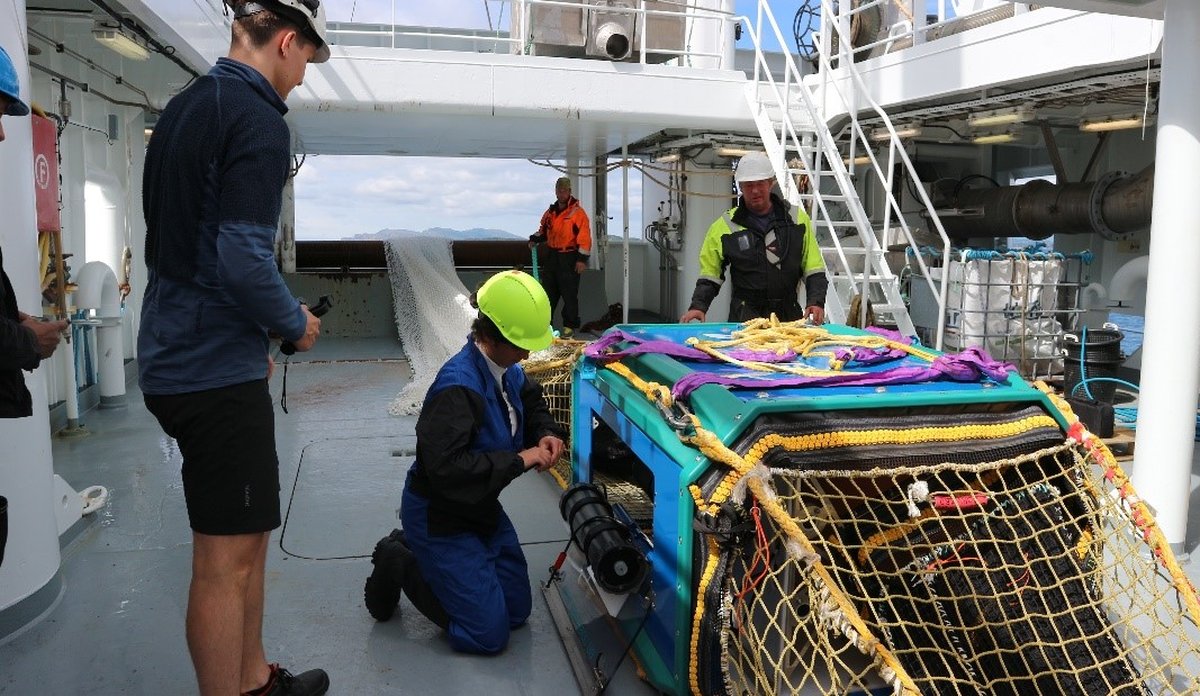
CRIMAC August highlight
Modelling
CRIMAC collaborates with an international group of scientists to validate and share code for acoustic simulations of frequency dependent backscattering from fish and zooplankton through the ICES Workshop on Acoustic Modelling1. An initial workshop was held in April, and the group works to establish the validity of a range of common models and implementations.
Broadband signal processing
A python library is being developed that reproduces the EK80 signal processing. The work is based on Andersen et al. (2021) and will aid researchers and students in understanding the steps involved in broadband echosounder signal processing. The library will also form the basis for further research and development in broadband echosounder signal processing.
In situ measurements
The frequency spectrum from sandeel schools have been measured with broadband echo sounders, 38, 70, 120 and 200 kHz (Figure 1). We are working on extracting information like size, behaviour and species classification. We are currently working on different algorithms to achieve this. We also used our kayak drone for concurrent observations from an autonomous platform.
Figure 1: Sand Eel broad band frequency response.
Net pen measurements
Figure 2: Net pen broad band observations.
Masterstudents Kristin Utne Berg and Maren Rong have measured the broadband echo spectrum from salmon in submerged net pens (Figure 2). Submerged net pens are designed to restrict salmon to the sea lice layer at the surface. The salmon was deprived from air access, and the change in echo spectrum was monitored over 3.5 weeks (Figure 3). The objective is to find signals in the data that indicates lack of access to air, and then trigger an alarm.
Figure 3: Echogram from the beginning and end of the experiment. The change in target strength between the start and the end of the experiment is clearly visible.
Net sampling
The Deep Vision system is used to image the organisms that enter the cod end of the trawl. For demersal trawling, the sand cloud makes imaging challenging, and we are developing a set up that lifts the imaging unit above the sand cloud. We are also developing a simple stereo-camera frame with GoPros and extra light for length measurement and behavioural studies of fish in trawls and other platforms.A two-day survey was carried out in the end of June to test handling of Deep Vision on board MS Vendla, preliminary investigations of DV on catch efficiency and trawl geometry and teaching practical use of the system for IMR employees by Scantrol Deep vision.Taraneh Westergerling is soon submitting her MSc thesis with the title: A comparison of an in-trawl camera system to acoustic and catch results for small pelagic and mesopelagic fish species. Her supervisor is Shale Rosen (Figure 4).
Figure 4: Taraneh is getting the Deep Vision ready for deployment in the Multpelt trawl with instructions from Eirik Svoren Osborg from Scantrol Deep Vision.
Data processing
A data processing pipeline for using machine learning methods on echosounder data have been developed2. Each step in the process can be run independently in a container (Docker), and the code is openly available under an open source license.
We have also tested different pre-processing/gridding strategies to fit acoustic data to the machine learning libraries. Regridding in range and keeping ping resolution performs best. A report from a workshop on machine learning and echosounder data have been published3. A challenge with deep learning models is lack of training data, and a semi supervised approach for training a deep learning classifier on acoustic data have been developed. The method can successfully train a network with only 10% percent of the training data.
Autonomous vehicles
Methods for using autonomous vehicles to support our surveys are being developed. Sakura Komiyama completed her master thesis on the spatiotemporal distribution of sand eel from data recorded from Saildrones4, and we have another student working data from the Sprat survey.
New sensors
Data from herring layers using the Simrad EC150-3C (ADCP) have been collected, and methods to estimate fish migration behaviour and vessel avoidance are being developed.
Calibration methods on broad banded transducers are being further developed, both for continuous wave (CW) and frequency modulated (FM) modes, including testing different spheres and bindings and exploring bottom calibrations.
Outreach
An essay on smart fishing in Norway have been published (Handegard et al., 2021).
References
Andersen, L. N., Chu, D., Heimvoll, H., Korneliussen, R., Macaulay, G. J., and Ona, E. 2021. Quantitative processing of broadband data as implemented in a scientific splitbeam echosounder. arXiv:2104.07248 [eess]. http://arxiv.org/abs/2104.07248 (Accessed 10 May 2021).
Handegard, N. O., Algroy, T., Eikvil, L., Hammersland, H., Tenningen, M., and Ona, E. 2021. Smart Fisheries in Norway: Partnership between Science, Technology, and the Fishing Sector. Journal of Ocean Technology, 16.
1) https://www.ices.dk/community/groups/Pages/WKABM.aspx
2) https://github.com/CRIMAC-WP4-Machine-learning/CRIMAC-pipeline
3) https://www.hi.no/hi/nettrapporter/rapport-fra-havforskningen-en-2021-25
4) https://hinnsiden.no/organisasjon/marin_okosystemakustikk/Sider/Mastergradseksamen-p%C3%A5-%C3%B8kosystemoverv%C3%A5kning-med-seildroner.aspx