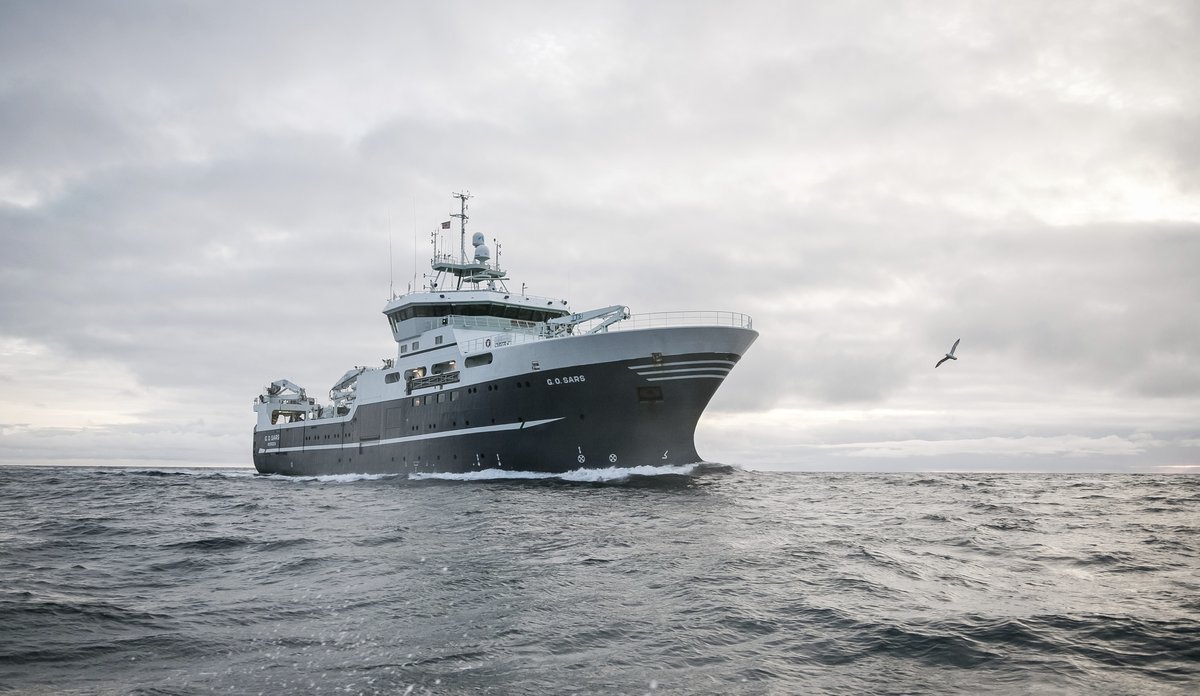
CRIMAC October highlights
State of the art
An important activity in the center is to keep up to date on state of the art within the field. A Zotero collection have been established to maintain key papers within the field. The collection is organized in broad band acoustics, acoustic modelling, sampling, and acoustic target classification.
Figure 1. The CRIMAC Zotero library.
Essay on machine learning + Marine science
We have written an essay on “Machine Learning + Marine Science: Critical Role of Partnerships in Norway” where we review the collaborations between marine science organizations in Norway (Handegard et al., 2021).
Limited amounts of training data
A main bottleneck in training deep learning algorithms on acoustic data is the lack of training data. In collaboration with the COGMAR project and the Visual Intelligence SFI, we have developed a semi-supervised method to detect Sand requiring only 10% of the labels compared to the earlier models (Choi et al., 2021).
Figure 2. Predictions of Sand eel and other species. From Choi et al. (2021), their Figure 3 (CC-BY 4.0).
New paper: The effect of shear-viscosity on resonance and target strength of mesopelagic fish
The target strength is required to estimate the abundance of mesopelagic fish. In this paper flesh shear viscosity is estimated, which is a key parameter for target strength, from in-situ broadband backscattering measurement (Khodabandeloo et al., 2021).
Figure 3. (left) Different swimbladder shapes. (right) the computational domain.
Calibration and data collection of broad band data from fishing vessels FF Libas
CRIMAC scientists tested, checked, and calibrated the Simrad EK80 echosounders mounted on the FV Libas. LIBAS is owned by Liegruppen, which is a partner in CRIMAC, and the crew were most accommodating both in skilful ship operation as well as providing enjoyable working conditions. The vessel will be used by CRIMAC to obtain echo spectrum response data on a range of fishes, like herring, mackerel, blue whiting, sandeel, cod, haddock, and redfish (Sebastes).
Figure 4. Calibration of Libas.
Collecting broad band data from fishing vessels
In September CRIMAC scientists joined the FF Libas during fishing operations and collected (calibrated) broad band data on Mackerel.
Figure 5. Broad banded echosounder marks on Mackerel shoals recorded off the coast of Norway.
Update the pipeline
The CRIMAC pipeline is a collection of processing steps for processing acoustic data for the CRIMAC project. As a part of this process CRIMAC scientists have been working with Rick Towler at NOAA on python software to read and process Simrad EK 80 data. The code can be found at Github: https://github.com/CI-CMG/pyEcholab
Figure 6. The CRIMAC pipeline.
CRIMAC survey on board GO Sars 1. – 21. November
We are finalising the preparations for a CRIMAC survey in the Barents Sea in November where the target is cod and other demersal fish. The crew consists of IMR scientists, partners from Kongsberg Maritime, Scantrol and NORCE as well as two of our PhD students from UiB. The aims of the survey are to gain better understanding of the broadband acoustic properties of demersal fish and other organisms and to develop image-based sampling methods in trawls near bottom. The survey also provides an opportunity for the industry partners to test and develop their instruments and methods for scientific and commercial purposes.
References
Choi, C., Kampffmeyer, M., Handegard, N. O., Salberg, A.-B., Brautaset, O., Eikvil, L., and Jenssen, R. 2021. Semi-supervised target classification in multi-frequency echosounder data. ICES Journal of Marine Science. https://doi.org/10.1093/icesjms/fsab140 (Accessed 12 August 2021).
Handegard, N. O., Eikvil, L., Jenssen, R., Kampffmeyer, M., Salberg, A.-B., and Malde, K. 2021. Machine Learning + Marine Science: Critical Role of Partnerships in Norway. The Journal of Ocean Technology, 16. https://www.thejot.net/current-issue/ (Accessed 6 October 2021).
Khodabandeloo, B., Agersted, M. D., Klevjer, T. A., Pedersen, G., and Melle, W. 2021. Mesopelagic flesh shear viscosity estimation from in situ broadband backscattering measurements by a viscous–elastic model inversion. ICES Journal of Marine Science. https://doi.org/10.1093/icesjms/fsab183 (Accessed 25 October 2021).